AI HAS ARRIVED
BY IAN DOIG • GRAPHIC COURTESY OF OLDS COLLEGE
Artificial intelligence (AI) is quietly revolutionizing processes that underpin sectors from astronomy and health care to manufacturing and entertainment. Research and development of AI in agriculture and agri-food is likewise revolutionary, with products now available or galloping toward commercial release.
Olds College of Agriculture and Technology is a leader in digital ag tech research. With the aid of machine learning (ML) and AI, its HyperLayer Data Concept project works to harness big data to assist farm production. Herman Simons, the College’s smart ag applied research manager, assisted in a recently concluded four-year HyperLayer initiative that studied the potential use of AI to provide agronomic advice. College researchers produced multiple layers of data for a series of crop fields. This included the extensive collection and analysis of soil samples, yield data and satellite and drone imagery. With substantial computing power, the project methodically built an algorithm to predict soil levels of available N-P-K and organic matter. “It takes a lot of time, but as long as you keep adding information it slowly but surely improves the outcome,” said Simons. For instance, the project has drawn a strong link between organic matter and nitrogen availability, but parallels with phosphorous and potassium require more work.
AI is a very powerful data management tool well suited to tackle the highly variable nature of farming, said Simons. “Data is the way forward for agriculture. As we gather more data, we become more knowledgeable about AI and how to implement machine learning.” As an example, he noted California farmers now use AI to schedule irrigation more efficiently and make better use of limited water. “You’ll see more and more AI and ML being implemented to make better decisions. There’s very little impact on farmers yet, but it’s going to change how we do things.”
“The algorithm will improve as we work on this over time.” —Greg Stewart
IN THE WEEDS WITH AI
Geco Strategic Weed Management has built two AI-equipped digital tools intended to improve weed management. CEO Greg Stewart has worked as a control engineer to improve digital and automated processes in the manufacturing and oil and gas industries. In recent years, he noticed the rise of data technology in agriculture and liked the prospect of helping farmers tackle uncertainty and feed the world.
In 2019, he joined Ecoation, an AI-driven greenhouse software company. Turning to broadacre crops, he launched Geco Engineering. With data sources such as satellite and drone imagery and precision ag platforms, the company creates predictive weed maps for farmers and agronomists. Its two products have been available since August 2023 and as of this spring it had 50 farm clients.
The Geco weed prediction system employs AI to model the activity of a weed population across five years. It considers weed location and biological details, cropping history and soil type. The system uses this to disrupt the weed’s lifecycle with the aid of prescription maps and a choice of three levels of management aggressiveness. The farmer uploads the preferred map to their granular applicator, seed drill or sprayer. The system also generates a five-year weed history report that can be used as a further decisionmaking resource.
“Many customers use our predictive maps to target soilapplied, residual herbicide on the weed hot spots,” said Stewart. These products can be effective as their modes of action differ from foliar chemistries. They are also expensive, so targeting established hot spots rather than blanket application is cost effective. Stewart used the ballpark comparison of $30 per acre versus $10 with a targeted approach. A farmer can also choose to fight hot spots with a non-chemical practice such as increased seeding rate. Stewart noted the extreme example of a client who seeded corn into weed hot spots in canola, which pushed those money-losing acres into the black.
The weed prediction system can complement the use of an optical spot sprayer, another emerging technology, said Stewart. The sprayer collects data that captures weed emergence on a given day, and this can be used by the prediction system to sharpen its long-term modelling. In turn, Geco’s AI models can predict the volume of chemistry the sprayer will require.
With the use of slightly different data, Geco also offers a herbicide resistance early detection service. Historically, weed resistance has been identified at about 30 per cent prevalence, said Stewart. The tool models weed behaviour to earlier identify and map small patches that behave abnormally. As well, a farmer can scale a suspected resistance problem down from, say, a 10,000-acre scouting chore to more manageable five- to 10-square-metre patches. If this precise scouting finds signs such as plants older than the last spray application, management strategy can pivot accordingly. Sampling these sites for lab analysis also increases the likelihood resistant weeds will be found before they multiply exponentially.
Having gotten its start on the Prairies, Geco is now exposing its system to as many crop, geography and weed combinations as possible, said Stewart. This includes Eastern Canada and the U.S. Midwest. “The algorithm will improve as we work on this over time.”
Hinting at the broader potential of AI to enhance agriculture, is Geco’s “frictionless adoption.” The tools do not require new equipment or practices, and a farmer can employ a prescription map within a day of signing up. With the use of AI, big data and equipment such as optical spot sprayers on the rise, five years from now, weed control will approach its optimal potential, Stewart believes. “We’re on a really interesting and exciting journey.”
WORK IN PROGRESS
Formed in 2019 and headquartered in Edmonton, the Canadian Agri-Food Automation and Intelligence Network (CAAIN) assists in the development of data-driven farm technologies. These include the use of automation, robotics and AI, which is a component of most CAAIN projects.
In its work with small and medium sized tech companies, CAAIN’s goal is to serve farmers, said CEO Darrell Petras. “AI is being applied to decisionmaking to support three general drivers on the farm: profitability, sustainability and productivity.”
Though not risk-averse by nature, multiple challenges such as variable weather and fluctuation of input and commodity prices have stretched the capacity of farmers to employ new technologies, said Petras. CAAIN works to minimize adoption risk and incentivize the use of AI by focusing on highly promising projects. This process includes co-operation with Canadian smart farms. Through field-scale research, these facilities demonstrate and validate new technologies for use in individual geographic regions. Petras admitted positive research results can’t always convince farmers to employ a product. While AI-driven technology development has caught fire, adoption has been slow.
Despite some headwind, the power and promise of AI has given its development undeniable momentum. The diversity of CAAIN projects that incorporate AI illustrates the value inherent in the technology, said Petras. It can enable the use of digital data for real-time decision-making in ways the industry may soon take for granted.
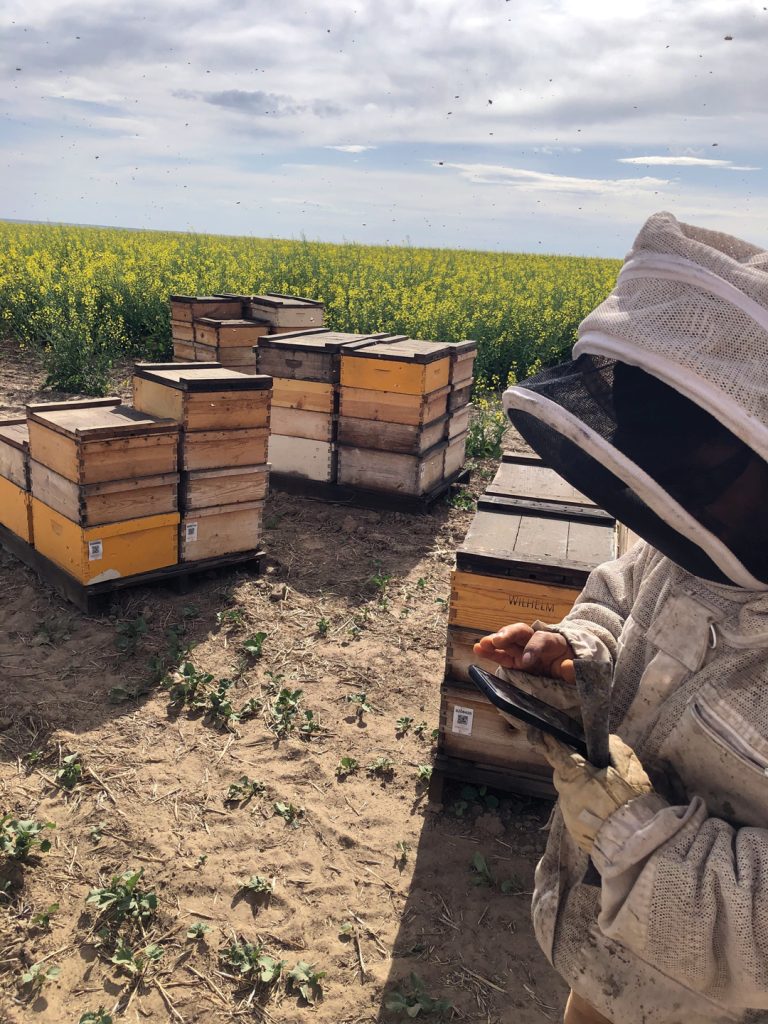
BE ALL THAT YOU CAN BEE
As an industrial design student in Montreal, Marc-André Roberge took up urban beekeeping. “In French they say ‘getting the sting for it’—pun intended. I really fell in love with beekeeping.” He found it tricky to keep the honey producing insects alive and healthy, and discovered his hobby hive experience paralleled that of commercial operations. Large-scale beekeepers face numerous management challenges such as climate change, pests and diseases that lead to annual bee die-offs.
To help beekeepers manage their operations more efficiently and profitably, Roberge co-founded Nectar Technologies and set to work building the BeeTrack traceability platform. Working extensively with Canadian and U.S. beekeepers, Nectar refined the tool and launched it commercially in 2020. The company now has a staff of 15 and BeeTrack is used in honey production and pollination services for flowering crops across Canada and the U.S.
To further sweeten the prescriptive abilities of the service, BeeTrack is working with CAAIN on an AI component. The project has involved the collection of data from multiple bee operations to train the AI. The beekeeper inputs hive information such as the amount of honey produced and pollination duty schedules. GPS co-ordinates enable hive traceability and are layered upon third-party data that includes satellite imagery. This can be used to determine a hive’s exposure to crops, chemistries and air quality, all of which informs care, feeding and the prediction of winter survival rates.
“We build best management practices in a data-driven way,” said Roberge. “The AI doesn’t make decisions for the beekeeper but augments their capability to make the best decisions possible to run a more profitable business.”
Nectar began the launch of BeeTrack’s AI enhancements in January with a feature that predicts hive survival over winter. It will continue to incorporate additional AI capabilities as they are developed.
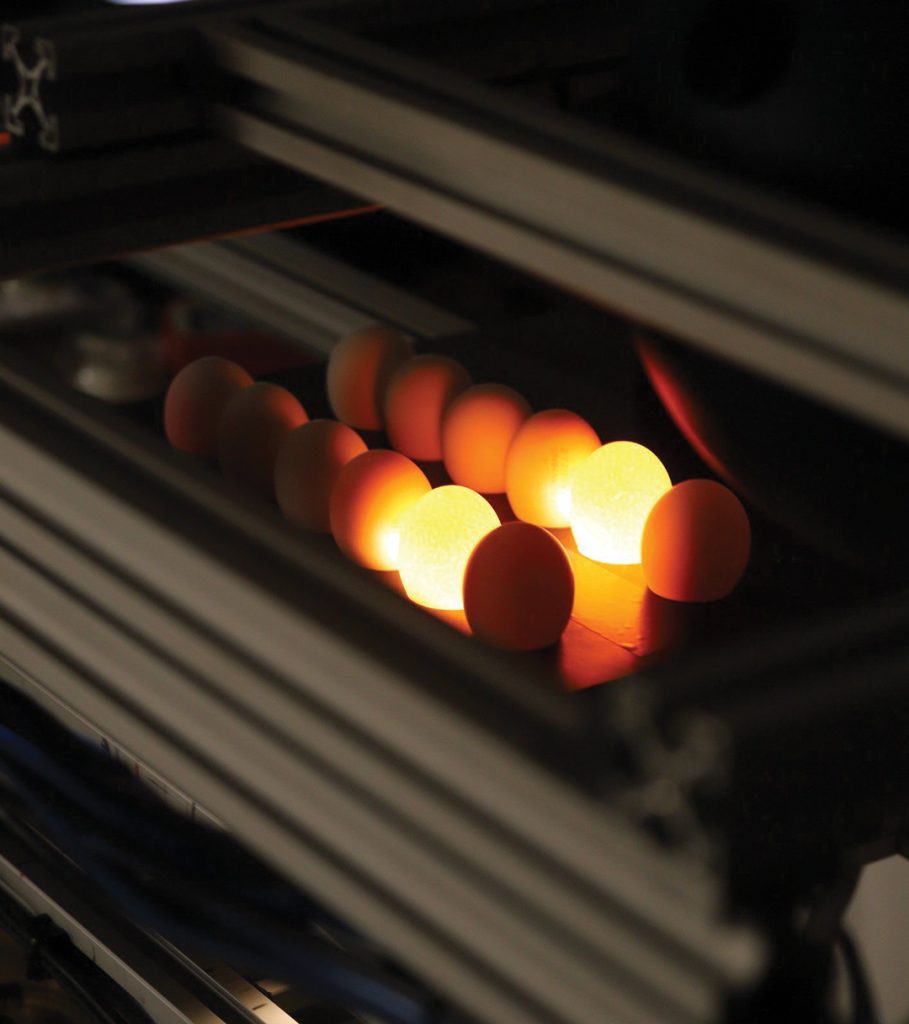
A WINNER IN THE SHELL GAME
Now used in satellite crop analysis, hyperspectral imaging is a photographic process that captures the visible and invisible aspects of the light spectrum, including infrared. More than 15 years ago, food engineer Michael Ngadi suspected it could be used in food quality assessment and began to experiment with a hyperspectral camera. In a literal lightbulb revelation, Ngadi realized it could be used in chicken hatcheries. As a McGill University professor and CEO of MatrixSpec Solutions, he has developed the idea with the addition of AI and assistance from CAAIN.
He and his team worked with an industrial design company to create a special lightbulb to illuminate the eggs for the camera. This Hyper-Eye system can assess an egg’s physical and chemical composition. “You can collect humongous data about the egg,” said Ngadi. “We train the computer to recognize and assess that data.” In hatchery operations it can determine the quality, fertility and gender of a pre-incubated egg.
The innovation has huge implications for hatcheries, as they require only female birds. To cull male eggs rather than day-old chicks would eliminate 21 days of incubation time and euthanasia of these birds. Worldwide, approximately seven billion chicks are destroyed annually. The Hyper-Eye system can save energy and expense and have a positive impact on the environment, said Ngadi. “Every day we come in to work excited to be part of this solution to a worldwide problem.”
Staffing is the downside of working at the cutting edge of AI, said Ngadi. MatrixSpec has drawn from McGill and the University of Montreal, which constitute a hub of AI activity. However, he noted many Canadian AI professionals do not operate in the ag and food space, and the industry competes for talent with Google, Amazon and the aerospace sector.
The Hyper-Eye is now undergoing intensive laboratory testing that will be followed by pilot testing in hatcheries. Ngadi predicts it will be marketable in a year or two.
COOLED TO PERFECTION
An agrifood professional with expertise in finance, meat science and process engineering, Cameron Bergen spent three years assisting in the construction of a state-of-the-art, commercial food production facility. The project demanded intense, technological problem-solving and inspired him to help more food industry companies reinvent and streamline their processes. In 2020, he co-founded mode40, which develops high-tech manufacturing processes and technological operating solutions for agri-food clients.
Bergen and his workmates identified problems they could potentially solve and found a glaring example in the meat processing industry. Carcass cooling has traditionally been lightly managed owing to general manpower scarcity in the food and beverage sector and the high cost of custom-built equipment. With help from CAAIN, mode40 is developing the Meat Quality Management (MQM) system, which uses AI to produce recommendations for real-time management of carcass cooling. The system’s sensor array collects multiple data points on conditions within the cooler. It correlates with carcass weight to produce handling recommendations. The novel system is intended to improve meat quality, cutability and food safety. Bergen believes it will also produce cost savings and increased profit without the need for costly infrastructure investment.
“The data collection, mathematics and thermodynamics issues are complex, but once those are solved, it’s easy to rinse, lather, repeat,” said Bergen. Proven to work in carcass cooling, the framework should apply to numerous similar processes such as cooking, marinating, slicing and smoking of meat products. He emphasized AI-based tools such as MQM are not the stuff of an imagined future. “AI is solving real-world problems today.”
The system is now being validated in 10 meat plants in Canada and the U.S. Bergen is confident this pilot stage will prove it suitable for small-scale abattoirs and scalable for large facilities.
Comments